Is That Ethical?
Episode 16: Is That Ethical?
===
Morgan VanDerLeest: [00:00:00] Hey everyone and welcome back to the PDD podcast. Today's episode has a distinguishing trait that makes it a bit different from the ones that we've done so far. It tackles a topic that, unlike most things that we've covered, doesn't have a widely accepted ground truth when it comes to what's expected of an engineering leader.
We're talking about the dicey and often gray area of ethics in engineering leadership. On the surface, our listeners question today didn't specifically ask about ethics. It was looking more for deterministic guidance, but we're pretty sure that once you hear it, you'll understand why we took it in this direction.
Eddie, why don't you do the honors?
Eddie Flaisler: Y I am head of engineering at a startup that just closed at Series A. We've been around for about three years, and for most of that time, our value proposition didn't quite land. Luckily, once generative AI solutions hit the market, we managed to pivot and finally got traction. Our tech feels relevant and users and investors are excited. But here's the catch:
to deliver what we need, our AI requires broad, unsupervised access to user's personal documents. [00:01:00] Technically, they've consented, but I doubt they grasp the scale of what we're storing or how some of that overwhelming context ends up influencing our systems behavior in unpredictable ways. I've raised this with our CEO, who waved it off.
They said that as long as our security holds and Legal is fine with it, we're in the clear. That's probably true, but I can't shake the feeling that we're crossing a line our users wouldn't knowingly step over.
At the same time, I believe in the mission and I like my job. What would you do if you were in my shoes? How can I make a stronger case as a CEO without sounding alarmist or naive?
Morgan VanDerLeest: Oh man. Eddie, I don't know about you, but I don't think we can give this person what they're asking for. This is so nuanced. I'm not even sure I would know how to approach it.
Eddie Flaisler: Well, we're in the same boat. I think we can agree it would be outright negligent to hand this person a set of talking points without knowing the full picture or what's at stake. But maybe what we can do is offer them a lens they can use to evaluate the situation.
Something to help them [00:02:00] make sense of it and arrive at a decision they feel comfortable standing behind.
Morgan VanDerLeest: I am good with that approach. Cue the intro. Let's do this.
I am Morgan.
Eddie Flaisler: And I am Eddie.
Morgan VanDerLeest: Eddie was my boss.
Eddie Flaisler: Yes.
Morgan VanDerLeest: And this is PDD: People Driven Development.
Eddie Flaisler: You know, Morgan, I don't think you've ever been so quick as you are today to say you don't know. What about this question has you so concerned?
Morgan VanDerLeest: Well, I don't think it's debatable that the last couple of years have brought some very significant and accelerated shifts socially, economically, politically, technologically. I can't think of many assumptions from five years ago that still hold true. And I think what such shift tends to do is reshape, at least in some aspects, our definition of right and wrong.
Especially within the context of corporate life. You know how important privacy is to me, but is it more important to decide for consenting users what is and isn't too much or to solve a real problem with the help of new technology and generate some jobs along the way? It might sound like I'm leaning one way or the other, but the truth is I'm not actually [00:03:00] sure.
And to be honest, I hate the fact that I'm not sure.
Eddie Flaisler: I totally hear you on that, and this actually raises a deeper question. You know, one of my favorite things to say on this show is that being an effective leader is all about alignment between you and your superiors. But is there a silent part to that alignment, which is, what is it exactly you're aligning on?
Like, sure, good for you if you're aligned with your boss on doing something that undermines human rights, doesn't make it okay.
Morgan VanDerLeest: So you do agree that there needs to be some code of ethics governing it all?
Eddie Flaisler: I think ethics is a really good way of looking at it because the study of ethics is meant to answer a question that is very relevant to our listener. Are there actions that are categorically good or categorically bad, irrespective of their outcomes or consequences? Now, if you think about it, me bringing ethics into the conversation defined that way should be a red flag for listeners.
They're like, wait a second. I'm here because I wanna do right by my organization. And right is going to be measured by the outcome, not by whether the action shows up in some [00:04:00] theoretical list someone made. But here's the thing, Morgan: ethics doesn't just categorize things as right or wrong.
It does so through the lens of whether those actions contribute to the wellbeing and flourishing of most people in a given situation, and that, I would argue is a lot more tangible.
Morgan VanDerLeest: I see where you're going with this and come to think of it, it actually simplifies how to get to the right answer in questions like the one our listener raised, which might seem on the surface, like just a matter of personal opinion. I'm suddenly realizing there are existing frameworks for that, like the code of ethics from uh, what is that?
The IEEE, ACM, and what's the other one?
Eddie Flaisler: IFIP, right? These three organizations have done a ton of work on codes of ethic in professional practice.
IFIP has this incredibly thorough code of ethics and professional conduct, but IEEE and ACM have really gone all in. Over the years, they've released a whole range of publications, some separate, some joint, and these publications cover everything from how we treat each other at work, to what [00:05:00] responsible software engineering looks like, to mindful practices around autonomous and intelligent systems.
Morgan VanDerLeest: To give our listeners some context. IEEE is a global organization of about half a million engineers and technologists. They've been around since the 1960s and are widely seen as the defacto authority when it comes to engineering standards. For example, they created and maintained the 802.11 standards, which are basically why we all have wifi.
Their mission is pretty cool too. Advancing technology for the benefit of humanity. That's awesome. ACM is the main global association for people in computing. It's been around since the late forties and operates in nearly 200 countries. It plays a big role in shaping how we think about computing as a science and a profession.
They've sponsored and promoted a lot of the foundational work in algorithms and programming languages, which gave tech the leverage it has today. IFIP is kind of the global umbrella for national tech societies. Together, they represent over a half a million IT professionals across 40 countries. It was set up back in 1960 with the support of UNESCO and it's been focused [00:06:00] on bigger picture stuff ever since.
Like tech policy ethics and making sure digital systems serve the public good. There's some serious folks in there.
Eddie Flaisler: By the way, before we move on, I have to call out the work being done by the EU and UK governments on data ethics and trustworthy ai.
We've already got more than enough on our plate today with reflections on the work of the three organizations we mentioned, but to our listeners, you should definitely look into these European initiatives. Some truly eye-opening work.
Morgan VanDerLeest: Okay, Eddie, as you said, lot of ground to cover. How do you recommend we approach this?
Eddie Flaisler: Well, when I first found out about all these resources, I was pretty shocked with how insanely large the body of work around ethics and technology is. And I'm not just talking about what these organizations compiled directly, but also about derivatives and research publications based off their work. It seems to go on and on.
I think the most effective way to review it would be to discuss the common themes.
Morgan VanDerLeest: Go for it.
Eddie Flaisler: Okay, so there are essentially four goals that are actively being pursued across [00:07:00] all the work I've seen on ethics in tech. Here they are. One, protecting people from physical and emotional harm. Two, treating people fairly.
Three, enabling people to understand and challenge decisions made for them. And four, safeguarding people's rights and freedoms.
Morgan VanDerLeest: I'll repeat those. 'cause they're important. Protecting people from physical and emotional harm, treating people fairly, enabling people to understand and challenge decisions made for them, and safeguarding people's rights and freedoms.
Eddie Flaisler: That's right. And I want you to notice that in describing these goals, I didn't use words like privacy or governance or auditability, and that's very intentional. Because if there's one thing we learn as engineering leaders in a business, it's that you don't get things done by stating the means. You get them done by stating the ends.
If I try to convince someone to rebuild a service because of tech debt or best practices, I may as well walk myself out of the room. But if I frame the conversation around the outcomes of the initiative, [00:08:00] then there are only two possibilities. One, I get to a yes. Two, I realize we're not aligned on the goals.
That is not to say that my audience doesn't have good reasons for that misalignment, but at least I know that the issue isn't, that I wasn't convincing enough. And when we're talking about something like ethics, which is so foundational to how we see the world and how good we feel about the system we're a part of, I think it's especially important to be crystal clear on what the desired end state looks like.
Morgan VanDerLeest: Shout out to previous episode where we talked about how valuable it is to be aligned with business outcomes. Any type of alignment that you're looking for, make sure that you're bringing those business outcomes into the conversation so that you can get either this, yes, or find your misaligned.
Eddie Flaisler: Absolutely.
Morgan VanDerLeest: So now let's take these four goals and use them as a framework for our discussion. Starting from the first one, which seems almost obvious, protecting people from physical and emotional harm.
Eddie Flaisler: That's a good one to start with, especially because in the context of a job, [00:09:00] it often gets reduced to how someone can make an impact in industries like military tech or surveillance. I don't actually think the most meaningful opportunity is to protect people from harm, come from trying to make good decisions within these industries.
I mean, sure there's a lot you can do from the inside, But by that point, the goal of the product is already set, and if you're on the team, you've made your choice. Plus, it's a pretty niche space. What's more worth digging into is how we as an engineering team, working on practically anything might approach the potential for misuse and the kinds of norm violations we don't always see coming
Morgan VanDerLeest: Interesting. Say more about that.
Eddie Flaisler: Not before we introduce the Tech Policy Lab.
The Tech Policy Lab at the University of Washington is an interdisciplinary collaboration established in 2013 by faculty from the School of Computer Science and Engineering, the Information School and the School of Law. Its stated mission is to enhance technology policy through research, education, and thought leadership, aiming to bridge the gap between technologists and [00:10:00] policy makers.
That all sounds great, but the reason I like them is because they have the best horror stories, and the one I'm thinking of is about gaslighting. This term doesn't require much introduction. Gaslighting is a very specific form of emotional abuse and mental manipulation.
It's meant to make someone doubt their own perception, memory, or even sanity. One common example, which gets called out a lot on social media, is when someone denies a fact to your face even though both people know it's true. But the original definition is actually more intense. It refers to quote, seeking to induce mental illness in another person by subtle and subversive changes to the target's environment.
True gaslighting has horrific medically documented consequences. It can trigger depression, anxiety disorders, complex PTSD, and even psychotic episodes in people who are more vulnerable to that kind of breakdown. In the Tech Policy Lab's example, they describe a recently [00:11:00] separated couple where the ex-boyfriend who still resentful, starts manipulating features of their formerly shared smart home.
His ex-girlfriend now lives there alone, but unbeknownst to her, he still has access to the controls. So he flickers the lights, he changes the thermostat, he rings the doorbell when no one is there. All subtle actions that leave her feeling unsafe and unsure of what's real anymore. She tries reaching out to the customer support.
But they have no activity record available. They just see the house user is logged in as expected.
Morgan VanDerLeest: That feels like a Black Mirror episode or something from like American Horror Stories. I don't like it. I'm probably gonna have nightmares about that. Awesome.
And I can think of more examples like a drone platform designed for deliveries that can be repurposed for unauthorized surveillance or even turned into a weapon in kamikaze style attacks. Or a fitness app that publicly shares your location, making it easy for some creep to track your movements.
And sometimes the harm isn't even malicious. It's just built in by [00:12:00] accident, like a medical device with an interface that is so unintuitive that clinicians end up entering the wrong dosage.
Eddie Flaisler: That's exactly right.
Morgan VanDerLeest: So we're clear on the problem statement, but what can the ENG leader do? We've said it before. There will always be something we haven't thought about.
Eddie Flaisler: There will be, but I think you'll find that preventative measures for so many misuse scenarios, including the ones we've both described, tend to fall into the same buckets. And frankly, these should probably be default requirements in almost every software product. I'm talking about granular user access, auditability, smart data retention, fail-safe defaults and bounded autonomy.
Morgan VanDerLeest: All right, Eddie. That is a very broad list and I definitely think we should double click on every item. But before we do, I want to call out what some listeners might already be thinking. #Purist. Good for you, man. But this huge list is basically like saying just do it all the right way. You know very well nobody has the time to do all of that.
Eddie Flaisler: I couldn't agree more. Not all of these capabilities should receive the [00:13:00] same level of investment, and honestly, this is one of the best examples of why risk assessment is such a critical part of our job as engineering leaders. You see, you're not expected to blindly address all of that. You just need to decide what's important for your use case.
Luckily, a lot of foundational work has already been done to help you decide what to address and how. There's a whole category of team exercises called foresight exercises where the team comes together to imagine how the product might actually be used. User story mapping is probably the most well known, but another one I really like is called Harms Modeling.
Microsoft has a great template for it. Just search Microsoft harms modeling. It's a very lightweight way to think through how things could go wrong or be misused. It's really just about asking the right questions during design, and this framework does a great job listing all of them.
Morgan VanDerLeest: That makes a lot more sense. Regarding the actual items on your list. I think it's best we talk through some examples to define what each of these [00:14:00] means.
Eddie Flaisler: Sure, let's start with auditability and granular user access. Think back to the gaslighting story I shared. It probably couldn't have gone as long as it did, or maybe wouldn't have happened at all if there had been a clear user friendly audit trail showing what actions were taken and by whom.
And if authorization was based on something more personal and easily verifiable like SSO and not a house user, she would've had a way to separate her login from his, and it would've been much easier for her to cut him off.
Morgan VanDerLeest: So side note, as a user, this is why you should not share logins. It's just a problem. But more importantly, this is why it's valuable for an engineering leader to have a voice in product and business concerns, like having this kind of information and this data, while it might seem superfluous in a product or business context without understanding.
This is incredibly valuable for the way that your users interact with your product, and to not have this information is honestly a glaring omission. All right, what's next?[00:15:00]
Eddie Flaisler: Smart data retention, or better yet, non lazy data retention. The absence of this is something I've been burned by personally.
Morgan VanDerLeest: As I would imagine from the way you said non lazy, I have my tea here. Please spill.
Eddie Flaisler: Well, if you must know, many years ago, I had a subscription to a music service, not the one you're thinking of. I canceled my subscription and closed my account back in 2021. A few months ago. I wake up in the middle of the night to a flood of email notifications. I check my phone and see that I've been welcomed back to this music service and notified that my credit card had been successfully charged.
I can't even begin to describe all the ways in which this is wrong. Talk about anomaly detection. In the middle of the night. An account that has been confirmed as closed four years ago is suddenly reinstated. No two factor authentication, no alerts. And apparently not only was my account still alive, but my credit card information was still on file four [00:16:00] years after I explicitly canceled and requested deletion.
And the biggest irony: there was something they did really well. I could not, for the life of me, find a way to contact support to report any of it. That part was secure.
Morgan VanDerLeest: Oh my God, Karen. I mean Eddie.
Eddie Flaisler: Go to hell.
Morgan VanDerLeest: But seriously, it's honestly tragic. That's such basic components of user experience are just thrown out the door or completely disregarded. In this case, that is terrifying. Although my bigger concern is why you have email notifications set to flood you in the middle of the night.
Eddie Flaisler: I, I actually thought about that only after it woke me up.
Morgan VanDerLeest: Life lessons. All right, let's talk to these last two. Fail safe defaults and bounded autonomy.
Eddie Flaisler: Yeah. Well, I think these two are essentially different flavors of the same thing.
Morgan VanDerLeest: Okay. I can see that. When the system enters a bad state or there isn't high enough confidence about what to do next, you want to default to the safest possible action. I. Even if that means disrupting service, it's like having an [00:17:00] autonomous vehicle safely stop at the curb when it's unsure what to do, or an EMR blocking a doctor from submitting an unusual dosage until additional confirmation is provided.
Eddie Flaisler: That's right, and I'm happy you brought up autonomous driving and medical systems in your examples, because this touches on a very important aspect of preventing harm and professional ethics in general, making sure you fully understand the core domain of the product or service you're working on.
Morgan VanDerLeest: Say more on core domain.
Eddie Flaisler: Core domain is a term domain driven design that basically defines the part of the system that is most critical and differentiating. And also where failure is the most disastrous.
One thing we tend to do in our fast-paced tech environment, especially during growth stage, is the path of least resistance to get to something working. So you focus on the happy path and edge cases are deprioritized for later, but depending on what you're building, edge cases can actually be a showstopper.
And in this case, standing up to potentially [00:18:00] less informed business stakeholders is ethical development.
Morgan VanDerLeest: As a parallel to some of our more technical folks, this reminds me of defensive coding. It's not about writing code to do the right thing. It's about writing code to prevent the wrong thing. It's a similar concept here. Be aware of your core domain and unintended consequences.
Eddie Flaisler: Totally, but I got us off track. Let's get back to bucketing fail safe defaults and bounded autonomy together.
Morgan VanDerLeest: Yeah, so to me, bounded autonomy simply means implementing fail safety faults by putting a human in the loop. I know that automation, intelligence and everything that makes our systems more efficient and independent has been a hallmark of the industry for decades. But it's important to remember, there are plenty of situations where having a human double check what the machine did isn't low tech or primitive software design.
It's just the right thing to do.
Eddie Flaisler: Well, as you like to tell me, say more.
Morgan VanDerLeest: Sure. We were talking about smart homes. Well, a very typical feature of those is climate control. To save energy, the system can turn off heating or cooling when it recognizes there's no movement in the house. [00:19:00] But what if you have a bedridden person who's sick or elderly, the wrong temperature can literally kill them.
Eddie Flaisler: Okay. That got dark really quickly, but you're spot on.
Morgan VanDerLeest: I'm just trying to take a page out of your book. Also, think of recommender systems. The ability to group items together based on similarity has been here long before the AI boom, and on paper it keeps getting better. But you really want to risk recommending diet products to someone buying recovery materials for an eating disorder. A person with the right background, and most importantly, sensibilities needs to regularly review the outputs of these systems.
Eddie Flaisler: I could not agree more.
Morgan VanDerLeest: I think it's time we moved on to the second goal pursued by the standardization of ethical development, treating people fairly. How do we tackle that?
Eddie Flaisler: I think the single most important concept to dive into when it comes to fairness is bias. This topic alone could fill an entire podcast series, but let's try to cover the key pieces here. Bias shows up in many forms, and there are several accepted ways to categorize it. For today's [00:20:00] conversation, we should probably focus on three main types, individual bias, organizational bias, and algorithmic bias.
Now, since this is a podcast on engineering management, it might seem like we should jump directly to algorithmic or maybe organizational bias, but the truth is you have to start from individual bias because the others are ultimately reflections of that.
Morgan VanDerLeest: This is actually not surprising at all. It's Conway's Law, right? Organizations who design systems are constrained to produce designs, which are copies of the communication structures of these organizations. So same idea. Individuals influence cultures and cultures influence what is being built.
Eddie Flaisler: That's a very clever way to look at it.
Morgan VanDerLeest: Thank you.
Eddie Flaisler: You are welcome.
Morgan VanDerLeest: So where do we start on individual bias?
Eddie Flaisler: Well, one of the best sources out there for understanding what bias actually looks like is Harvard's Project Implicit. They've been running this virtual lab for almost 30 years now. What they do is collect public data based on online trends and support research built on top of it.
Their work had a big [00:21:00] influence on Uber's cultural shift during my time there. Anyhow, it turns out that some of the more well-known folklores, so to speak about bias in the industry is actually rooted in deep research that project implicit supported. Like the tendency to implicitly associate men with careers and women with family, or the way mothers are often seen as less competent or less committed and less leadership oriented than non mothers.
There's also the implicit association of leadership intelligence and competence with white individuals, especially white men. While black professionals are more often associated with aggression or seen as less suited for manager roles.
Morgan VanDerLeest: That's what the tightrope bias refers to, right? Where non-white professionals have to constantly reprove their competence, and it still feels like never enough.
Eddie Flaisler: that's exactly right. Another commonly identified bias is against people with disabilities. I've personally witnessed some crazy examples where a very capable individual being wheelchair bound made people perceive them as [00:22:00] having an intellectual disability.
Research also backs common perceptions regarding ageism, LGBTQ plus individuals, especially presenting ones who cannot hide who they are, and even weight bias. So overweight individuals were found to be considered messy and low functioning, solely based on their appearance, which we know today often stems from far more than just negligent life habits.
Morgan VanDerLeest: And we've also touched in the past on bias against neurodivergent individuals.
Eddie Flaisler: Yeah. Which, at least in my mind, is one of the more complicated biases to address, because with ND individuals, it's not always clear what is that thing, which is different about the person. You just feel like it is. And you know Morgan, when you have a certain trait that bothers people, but they can't quite put their finger on what that is, that's exactly when they respond with microaggressions, with devaluation of your other strengths and the slow key resistance to including you in conversations or decision making.
Morgan VanDerLeest: Absolutely. Eddie, as you mentioned, there's a lot to dig into here, but how does this directly tie into our topic?
Eddie Flaisler: [00:23:00] Well, we're talking about fairness and we both know very well that a culture which does not promote fairness internally cannot produce something which promotes that externally.
Morgan VanDerLeest: Eddie, I hate to be the one saying this, but as inspirational as what you just said, sounds, I think we'll need to give people more concrete proof of that. Organizations are live organisms with their own inherent flaws. This evidently doesn't make them unfit to solve problems for others. Look around you.
Eddie Flaisler: Absolutely. So let's break this down. You know, bias is not a sign of character flaw. It's a natural tendency common to literally everyone which needs to be managed. This is especially true when you're an authority figure inside an organization. When you don't manage your own biases, there are several things you end up doing, whether you're cognizant of them or not.
One is you end up dismissing legitimate concerns raised by employees, and that includes ignoring or minimizing reports of serious misconduct. Two is that you can end up reframing your own or your peers and superiors [00:24:00] mistakes as failures of those who report to you.
Three is you often create emotional distress by constantly moving the goalpost or withholding support when it's actually needed and invalidating emotional reactions to unfair treatment. That's a big one. And of course, four, you keep hiring versions of yourself rather than building a team with truly complimentary strengths and perspectives.
Morgan VanDerLeest: Quick plug for introspection and having a psychologically safe environment so that if you're not introspecting it yourself, somebody else can check you on it so you're not running into these things on a regular basis.
Eddie Flaisler: 100%. And you know, the thing is that when you behave this way as a leader or allow your reports to behave like this, you are the organizational bias. We talk constantly about doing more with less and maximizing output from your engineers.
But here's the reality: when you allow these dismissive or manipulative behaviors to go unchecked, even unconsciously, you're building products that are guaranteed to fall [00:25:00] short. Why? Because the people building them who are the victims of these behaviors are not in any mental or emotional state to innovate with passion or focus on the customer.
Just because what you've shipped so far seems good doesn't mean it couldn't have been meaningfully better. And in this context, better doesn't just mean more features or cooler UX. It means that fairness, we are talking about algorithmic fairness, is an incredibly hard engineering problem. Not only mathematically, but also from a process standpoint.
Questioning the data you're using, catching edge cases, a lot of out of the box thinking goes into it. So you need an engaged team. And you know who always gets the short end of the stick when you're doing least resistance development to just get it done with? Accessibility users. Nobody ever thinks about them.
I was part of a team that developed optical character recognition, so basically image to text for some safety related purposes. I'm embarrassed to admit we were so consumed by wanting to show we delivered that it didn't even occur to us that people with [00:26:00] low vision who probably needed the safety feature the most, used their phone camera very differently to actually see what it is they're capturing.
So the recognition kept failing for them well after we went GA.
Morgan VanDerLeest: This is actually one of those reasons why I love to promote getting engineering involved earlier in the process and having a more collaborative planning process in general. Because if you have folks that are just, I hate to say it, but like CodeMonkey ticket pushers, you're not thinking about things like this. You're only thinking about, how can I complete the ticket that I have and not what is the experience someone is gonna have going through this? What are some things that we might have missed? What are the edge cases? Not just the edge cases of the code, but the edge cases of the actual usage.
Eddie Flaisler: No question about that.
Morgan VanDerLeest: Now, how about we dive in a bit into what algorithmic bias actually is, even assuming we have the right culture in place, as you yourself said, there's a lot to actually developing such minimally bias systems.
Eddie Flaisler: Makes sense. So first off, just for ease of search, if [00:27:00] people wanna learn more, we usually use the broader term algorithmic fairness. What that means is a state where automated decision making like credit scoring or job screening or content recommendation doesn't produce outcomes, which aren't actually justified just because someone belongs to a certain group.
You see what I mean? Now, do you wanna guess what the biggest challenge to achieving fairness is?
Morgan VanDerLeest: Probably what is fair?
Eddie Flaisler: That's right. How can you solve a problem that's so hard to define? Fairness is context dependent and culturally shaped. Believe it or not, there are even contradictory mathematical definitions for fairness. So you need to decide which one you go with. This is very noticeable in loan processing.
Imagine you're building a model to decide whether to approve a loan. So it's natural to ask how different groups of people, which you usually define by protected characteristics like race or gender or age, are treated by the system. So you wanna take a step back and say, what am I looking at to ensure fairness?
And if you think about it, there are [00:28:00] three things:
one, parity in outcomes, meaning you approve the same proportion of loans for applicants in different groups regardless of their repayment, likelihood.
Two is parity in errors. So you want the same false positive and false negative rates for everyone. And three is parity in meaning. If two people, regardless of group are given the same risk score, they should have the same actual likelihood of repaying the loan.
But the funny thing is that it's been proven you can't satisfy all three at the same time. Why? Because, for example, if one group has a higher historical repayment rate than another, a model trained on that data will naturally approve loans at different rates for each group, which inherently violates demographic parity. So in the real world, banks have to choose which definition of fairness to accept. And it's true for tech as well. You first need to decide what's important to you.
Morgan VanDerLeest: This very much sounds like one of those things that should be defined in your engineering values or in your company's [00:29:00] values. So you could say, these are the most important and why for us, so that individuals, managers, leaders within your organization can move forward knowing that we're at least all aligned in how we think about fairness.
Eddie Flaisler: A hundred percent.
Morgan VanDerLeest: Which actually begs the question, is there specific tooling for that?
Eddie Flaisler: Well, it really does depend on what you're building, but one of the most common frameworks used today is called Fairness Taxonomy, which is basically a structured way to define fairness according to different parameters and assess based on that custom definition.
This taxonomy forms the basis for two of the most widely used toolkits in the industry, which are IBM's AI Fairness 360 and Microsoft Fairlearn. What they do is help surface potential biases and disparities in model predictions. You can use them during model evaluation to run fairness audits. And I've even seen people integrate them into CICD pipelines to flag fairness regression, just like you would with failing tests.
They're especially valuable in regulated industries like finance and [00:30:00] healthcare or in high stakes domains like hiring or lending where legal, compliance and public trust can make or break your product.
Morgan VanDerLeest: Interesting. I do think that companies like OpenAI, Google and Anthropic rely heavily today on public survey to shape their models or. If to use the buzzwords reinforcement learning from human feedback.
You know, Google has tools like the perspective API, that scores text for toxicity. And Anthropic uses constitutional AI to guide Claude to follow a set of evolving ethical principles designed to reflect human values. That actually ties nicely into your point about the cultural context of fairness, because what's considered fair or acceptable behavior in one community might not be in another.
So these principles have to keep evolving alongside public norms.
Eddie Flaisler: No doubt about it.
Morgan VanDerLeest: Eddie, before we move on to the next goal, I wanna make sure we can summarize the takeaway here for people. The two biggest learnings for me here when it comes to pursuing fairness are that: one, I have to foster a culture which is conducive to attention to edge [00:31:00] cases and putting the customer front and center.
And two is that the foundation of every algorithmically fair system lies in the groundwork to decide how we as an organization define fairness, audit our data constantly to make sure it adheres to that and do the same for the output of the systems that ultimately makes the decisions.
Eddie Flaisler: Almost perfect. One more thing, probably more relevant to our next goal, but important here as well is transparency. Transparency is especially interesting. Remember how a few minutes ago I had to do some mental gymnastics to connect fair organizations to fair products? Well, with transparency, you don't need that.
It's already directly connected. Opaque team equals unfair model. Why? Because models aren't like deterministic code. There's no single line of code you can casually add a comment to, and suddenly it's clear how the decision is made. To build a transparent system, you need a team who's really good about documenting the entire modeling methodology, how the data set was collected, how it was cleaned, how it was [00:32:00] processed, what mathematical assumptions were made, why one machine learning algorithm was chosen over another.
And even maintain an audit log of model outputs. But when an engineering organization is opaque about how decisions are made, whether because of a culture of secrecy or just lack of process, it inherently means they're not producing this documentation. And that means they're fundamentally unequipped to understand, debug, or improve the model's performance or fairness.
You can't properly fix what you don't understand. So if you're not fostering a culture of transparency, you're essentially guaranteeing mediocre results.
Morgan VanDerLeest: Eddie. I love that. And I think your point about transparency is a good segue into the next goal, enabling people to understand and challenge decisions made for them. About this one in particular, I often wonder if we as an industry are thinking about it correctly.
Eddie Flaisler: Hashtag say more.
Morgan VanDerLeest: So one of the biggest areas of focus in machine learning, especially since the AI boom, has been explainability. So essentially being able to describe how the AI reached [00:33:00] the answer it gave to your question or request. And I completely agree that this is super important, but let's be honest.
The output of whatever thing people are now building to better explain how the model is thinking will probably not make a lot of sense to the general public. Yet they're the ones who most need to understand why a decision was made. Especially when that decision affects their lives. And even more importantly, they need a way to challenge or escalate when something seems wrong.
Didn't we just see this piece in the news last month about an insurance provider's AI model that denied claims wholesale, and then it turned out it had a 90% error rate. Even if God knows why, I'm gonna assume positive intent and an honest mistake. This perfectly exemplifies the need for scalable frameworks and tooling to efficiently incorporate feedback from users into the process.
How do you even get to 90% if you're not building in a vacuum?
Eddie Flaisler: Yeah, for sure. I had the same question. I think that, as you mentioned, there are two directions this naturally needs to go in. One is AI explainability, where the audience includes the [00:34:00] organization building the system, regulators overseeing it, and power users or third party developers who need to decide whether they can trust and integrate with it.
I think the amount of investment currently being put into this is definitely warranted. This is a really hard problem known as monosemanticity. The study of monosemanticity in machine learning is about getting to a point where you can profile a model's internal state, whether that's its memory or activations or some other operational data, and actually read from it clear distinct concepts in its thought process.
Things like the word banana or this sentence is a lie or python backdoor. My mental model for this, which may not be accurate but helps me think about it, is like mapping gene expressions to traits. I have hazel eyes. Why? Somewhere in my DNA is an expression pattern that explains that.
So similarly, you wanna be able to say, here's the feature that activates whenever the model thinks about deception, or this is where it [00:35:00] stores the idea of sarcasm. And much like with the human brain, it's incredibly hard to articulate the full thought process a model goes through to get to the answer you see. But that's exactly what we have to solve. Not necessarily, so the general public can interpret the output directly, but so we can trace how decisions are made, explain them, and not less important, catch early signs of reasoning that might lead to harmful outcomes. You see what I mean? You wanna know what it will output before it actually outputs something bad. This one is especially urgent because unsurprisingly, as AI systems improve, adversarial techniques are improving just as fast.
One of the biggest threats is something called prompt injection, where an attacker manipulates the model with seemingly harmless inputs, tricking it into things like exposing private data or explaining how to make a bomb or just otherwise weaponizing it.
And by the way, harmful behavior can even emerge without anyone trying to cause it. A model can cause harm simply [00:36:00] by being too nice. I've been really bothered over the last couple of days with one of the AI chat bots I use. It suddenly started telling me I was right all the time and praising every silly question or absurd claim I made.
It was funny at first, but eventually it made it useless for me. Sycophancy in AI models is much more dangerous than it seems. When models consistently agree with you to avoid conflict or appear helpful, they risk reinforcing false beliefs or enabling bad behavior or given a false sense of competence.
We've seen the echo chambers that social media algorithms created. Here, you don't even need others who think like you to convince yourself of something wrong. So it's really important to know what the model is going to say before it says it.
Morgan VanDerLeest: So what I'm hearing is that I can use this AI chat bot and I don't even have to deal with other people, and I can always feel good and right about myself. Please send me a link.
Eddie Flaisler: I know, right. My dream coming true.
Morgan VanDerLeest: But back to my original point, surely you agree there's definitely a gap in how we collect and act on user feedback. I don't even think this is a technology [00:37:00] problem as much as it is a process problem. Think about how it works with traditional systems. You've got humans making decisions, communicating those decisions in writing, and at least in theory, being available to answer questions over the phone, explain what happened, and more importantly, review and reverse a decision if your challenge makes a good case. That system is far from perfect, but at least it builds some mechanisms for accountability and correction. So now when we transition to AI based decisions, we can't just say, well, we still have those escalation workflows in place. In high volume, high stakes scenarios like insurance claims, immigration decisions or benefits eligibility. The main reason organizations introduce AI is to gain efficiency and process more requests faster. But that introduces the Jevons paradox, right? The more efficient you get at processing your requests, the more requests you end up processing, which means more decisions, more edge cases, and naturally more escalations, even if your AI is doing a decent job. So unless your escalation process actually scales along with your automation, you're just kicking a can [00:38:00] down the road.
And ironically, that might make things even worse than the human system you were trying to improve, because now not only are people affected by decisions they don't understand, but the pathway to challenge them is even more out of reach.
Eddie Flaisler: I do agree, and especially because as you mentioned, this is much less about cutting edge technology and more about intentional process design. I would have just one main takeaway for people when it comes to pursuing the goal of enabling people to understand and challenge decisions made for them.
Practice accountability in your organization and in your own work. The problem of feedback collection and escalations at scale has long been solved. There are road tested methods to improve models by incorporating feedback and there are dedicated teams doing for you as we speak, the work on AI explainability. All that's left for the engineering and product leaders using these models is to make customer impacting decisions thoughtfully to ensure these decisions align with the organization's core principles and values. Not to mention ensure the organization's principles and values actually mean [00:39:00] something and once done to fully own these decisions.
And course correct if they prove to be wrong. No way around it.
Morgan VanDerLeest: This is a great example of not needing to reinvent the wheel. A lot of these things have already been figured out for you. Lean into those. Yes, they may be best practices only for the last couple of months or couple of years, but these methods exist. Do your research ahead of time so that you're appropriately using the tools that are available to you.
Eddie, we're getting to the final part of our episode today, which covers the last goal, safeguarding people's rights and freedoms. I wanna blurt privacy, but I'm curious if you wanna make more connections here.
Eddie Flaisler: I do. Well, in a funny way, even though this goal is often discussed in the literature as its own category of work, and I don't think either of us or anyone in our circles would question its criticality. I almost see protecting rights and freedoms as kind of a kitchen sink goal. Think about it. Being treated fairly is a human right.
Being protected from harm is a human right. Understanding decisions that [00:40:00] impact you and being able to challenge them is, well, at least in non authoritarian regimes, a procedural and civic right? It's rooted in principles like due process and access to redress. That's why even though mentions of this goal in the literature typically focus on auditability and other forms of oversight of what is done to people, I would love to use the rest of our time today to talk about something broader, which is engineering for the greater good.
I think that's probably the type of work most closely associated with this goal.
Morgan VanDerLeest: Do you mean work streams like climate tech or engineers without borders?
Eddie Flaisler: Engineers Without Borders is, is that a thing?
Morgan VanDerLeest: Apparently.
Eddie Flaisler: Well, I'm sure they're doing God's work over there, but what I was thinking we could cover was what someone who isn't necessarily in a position or organization where they get to make clear positive impact just by the nature of their work, can still do for the greater good.
And you mentioned privacy. So let's start with design choices.
Morgan VanDerLeest: Go for it.
Eddie Flaisler: Our listeners' question today touches on a common challenge [00:41:00] faced by many companies that use AI to help businesses better understand and leverage their own data. And that is how do we store all that information without putting the customer's intellectual property at risk in the event of a data breach?
And we do need to keep track of it, right? Let's say we process one of their files into some unintelligible vector form, something only our model can meaningfully interpret, but then if we get notified that someone edited that file, how can we tell if it changed significantly in the way that would warrant reprocessing if we're not storing the original file?
I recently sat in on a design discussion where this issue was brushed off with just store the file, we have encryption. And I flagged that. People spend months grinding leetcode problems to prove they can think algorithmically. And I would love to see that same level of computer science rigor applied here.
Sim hash, given to us by God and Stanford’s Professor Moses Charikar, was designed for exactly this kind of situation. It's a type of hash that wouldn't just tell you if a file changed [00:42:00] by comparing the hash of the current file to that of the previous version, but also gives you a sense of how much it changed so you can decide if reprocessing is worth it without storing the file.
Claude and ChatGPT know all about it. We just have to care enough to ask.
Morgan VanDerLeest: That seems to be really indicative of the fact that in this era when there's just so much information broadly available, that it's much more important to be asking the right questions than it is to be having the right answers.
Eddie Flaisler: Totally.
Morgan VanDerLeest: Anyhow, I see where you're going with this. We should probably also call out carbon efficient computing, which by the way, I don't feel gets discussed often enough considering it usually comes bundled with lower cloud and data center costs. You save carbon emissions by reducing electricity usage. Electricity usage can be reduced by using fewer CPU cycles, consuming less memory and running workloads more efficiently.
But if all of that is true, then you're also spending less on compute, period.
Eddie Flaisler: Totally. That can be a win all around. You know, I recently saw a comparison between Golang and Rust as two competing languages for real time log processing pipeline.
It was kind of [00:43:00] shocking. They designed each implementation to play to the strengths of the language, and Rust ended up consistently using only 20% of the computer and memory that Golang did with respective reductions in cost in carbon emissions compared to the Go version. Now, don't get me wrong, Golang definitely has its merits.
It's easier to onboard. It's probably the best when it comes to concurrency. But the point is not going with Rust isn't an obvious call. It's a mature error safe language that gives you all the benefits of C and C++ without usual baggage. Awesome for this use case, you mostly need to pay the price of onboarding that is a little bit steeper. By the way, I also recently discovered an awesome website called Electricity Maps. It's super useful if you need to run jobs in the cloud, like data processing or batch analytics or machine learning training, and have some flexibility around where and when they run, which is sometimes the case with non-urgent or scheduled workloads.
What it does is shows you whether a region or even [00:44:00] a specific time of the day in that region, is cleaner, meaning a higher percentage of its electricity is coming from low carbon or renewable sources like wind, solar, and hydro rather than fossil fuels. So just by choosing a better time and location to run your jobs, you can meaningfully reduce carbon emissions without changing anything else.
Morgan VanDerLeest: I feel like it's the case that there's a lot of tooling nowadays that's making that easier and easier to be able to take better advantage of these things, which is awesome. I love to see that.
Eddie Flaisler: Absolutely. Anything else comes to mind, Morgan?
Morgan VanDerLeest: Yes. Please stop with the deceptive user experiences. Make your cancel buttons clearly labeled, not buried in a maze of confusing steps. Let's stop turning close icons into links that everything the user was trying to avoid. Let's ask permission to share people's data in plain language, not hidden behind technical jargon.
And let's just remember, the deceptive practices do much more damage than just momentary manipulation. They violate users' dignity. They take away their freedom to decide, and worst of all, they help normalize these cringey patterns across the [00:45:00] software industry.
Eddie Flaisler: Amen to that. Let's all just try to be good. And remember what Eddie always says, I did not go to grad school to end up at the center of a Federal Trade Commission complaint.
And on that positive note to the listeners, if you enjoy this, don't forget to share and subscribe on your podcast player of choice. We'd love to hear your feedback. Did anything resonate with you? More importantly, did we get anything completely wrong?
Let us know. Share your thoughts on today's conversation to People Driven Development, that's one word, peopledrivendevelopment@gmail.com. Or you can find us on X or Twitter @PDDpod. Bye everyone.
Morgan VanDerLeest: And if you're interested in a short book on "the things that Eddie always says", please shoot me an email. Cheers.
Creators and Guests
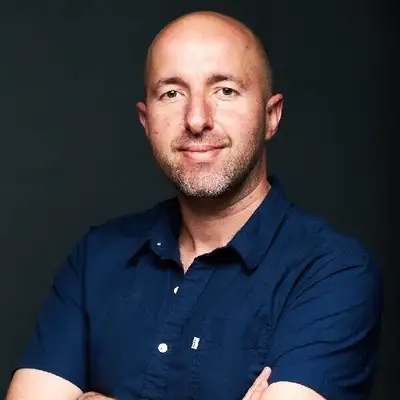
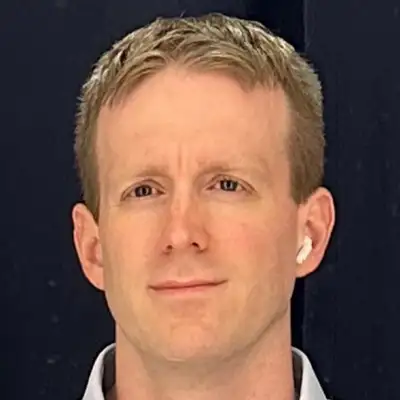
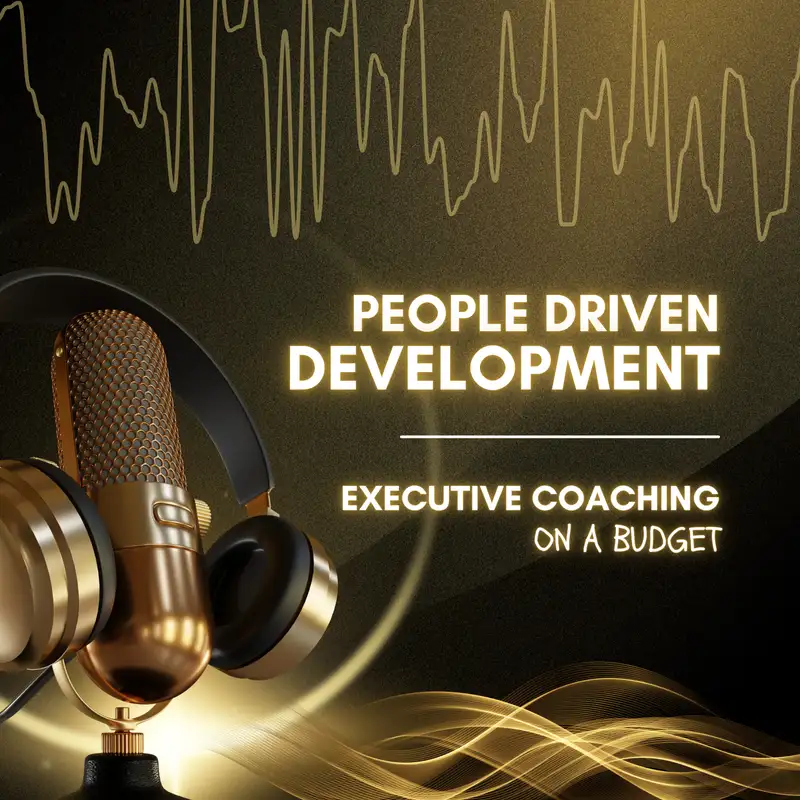